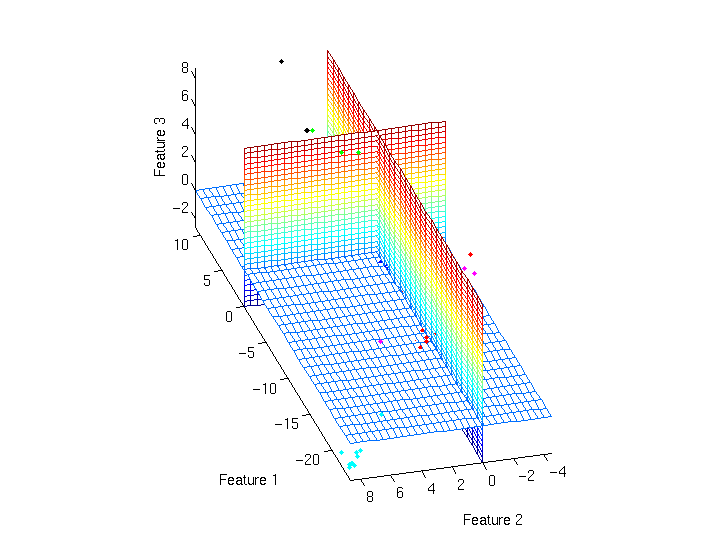
Nonlinear Principal Component Analysis Crack + [Mac/Win] In order to reduce dimensionality and to preserve the local features, a principal component analysis (PCA) method is proposed. For a dataset of m observations of n features, PCA is an unsupervised learning technique that describes the data set as a linear combination of a set of orthonormal bases. The principal components of the data are extracted by performing an eigen decomposition of the covariance matrix of the dataset. The first principal component has the largest eigenvalue, and the principal components are computed in descending order. The covariance matrix of the dataset is given by C = [ Σ ′ Σ ε Nonlinear Principal Component Analysis PC/Windows [Updated] The Nonlinear Principal Component Analysis Crack Free Download is a multi-layer perceptron that can be trained to learn the principal components (PCs) of input data. The learning of nonlinear principal components is performed by training with the nonlinear principal component analysis. The first principal component (PC1) should be able to represent 95% of the total variance in the data. The second PC should be able to represent the 99% of the remaining variance. The output of the network is a vector that describes the principal component. This vector is the unique input of the nearest neighbor classifier. Algorithm The data is fed to the PCA network. The input is put in a list and the first principal component is taken from this list. The total variance of the data is computed. The coefficient of variance of the data is computed. The variance of the first PC is computed and compared to the coefficient of variance of the data. The variance is stored in the first PC as well. This value is the principal component score. The coefficient of variance is stored in the second PC as well. This value is the principal component score. Step 4 is repeated until 95% of the total variance is stored in the first PC and 99% of the remaining variance is stored in the second PC. Training PCA networks for nonlinear principal components A nonlinear principal component is described by a weight vector. The training procedure to learn a nonlinear principal component requires first to define the architecture of the network and then define the training procedure. For learning the architecture of the network, the following options are available. Generic neuron units. Weight reset. Activation function. Scaling. PCA components vector. The weight is an activation that is used to compute the output. The weights are initialized with a random value between −1 and 1. To learn the network, the following options are available. Independent data. Multiple linear principal components. Automatic algorithm for learning. Multiple layer architecture. Different learning algorithms are available. In the architecture, the scaling of the weight can be constant or weight reset. Weight reset can be used if the weights are allowed to vary during training. Scaling allows the weight to increase or decrease, depending on the training progress. A nonlinear principal component is a single vector that represents 95% of the total variance. Learning multiple nonlinear principal components The learning of multiple nonlinear principal components can be performed using multiple layers. A number of successive principal components is learned until 95% of the total variance is described by the first PC and the last PC describes 99% of the remaining variance. This multi-layer learning can be used for learning multiple nonlinear principal components. The data is fed to the network. 1a423ce670 Nonlinear Principal Component Analysis Download This library is based on the keymacro algorithm, which is a c++ implementation of the MACRO (Method of Activating Conference) cipher (MACE) (Brown, D. G., McArdle, J., and van der Meulen, J. V. “A. M.”), which is a block cipher (in the sense that it operates on blocks of data) devised to be used in spread spectrum radio applications (e.g., cell phone communications). Keymacro was originally developed by J. V. van der Meulen of Stanford University as part of the development of the S-PACE (Spread Spectrum Positioning System) system. The MACRO was defined by Brown, et al. in 1983 and received more attention when it was rediscovered by Harada and Tsudik in 1998. Like many other block ciphers (e.g., RC4 and DES), the MACRO was first described in the literature in the form of a ciphertext. It has two ciphertext formats: rectangular and multi-column. For a given plaintext block, a MACRO ciphertext block is obtained by performing three basic operations: add, subtract, and modulo-2. The operation of the MACRO is a block cipher. The output of the MACRO, an encrypted block, depends on the plaintext block, the key, and a specific bit position (“offset”) within the key. Note that the key is a long bit string of fixed length; the key length is equal to the block size and the offset is a constant integer which controls the position within the key. The operation of the rectangular MACRO is as follows: 1. For each input plaintext block, compute a MACRO ciphertext block by performing a 3-term Fourier series expansion of the plaintext block and adding the Fourier series terms. (For a 3-term Fourier series expansion, use the algorithm described by R. van Stee of Carnegie Mellon University. The offset can be set to zero.) 2. XOR each plaintext block, with its corresponding ciphertext block. 3. XOR each XORed plaintext block with its corresponding XORed ciphertext block. 4. If the offset is non-zero, modulo-2 each of the What's New In? System Requirements For Nonlinear Principal Component Analysis: NOTE: As of v6.3 this mod has been superseded by the Pro-Tonpack, allowing you to carry two sets of gear. The main requirement for this mod is CPU and RAM, and if you have more than the minimum requirements you should go into the CPU and RAM settings in the options and change them to as high as you can and still have the game run smoothly. If you have high enough CPU and RAM, but if you are planning on modding the game, the mod also recommends an SSD. For the most
Related links:
Комментарии